Elasticsearch and AI: Revolutionizing Data Search and Analysis.
Elasticsearch, a distributed search and analytics engine, is often associated with powering searches on websites, applications, and other platforms that require fast, full-text searches. However, in combination with AI, Elasticsearch is transforming industries, from e-commerce to healthcare, by enhancing the capabilities of both search and analysis systems.
Let’s dive into how Elasticsearch and AI work together, what this partnership enables, and the benefits across various sectors.
1. Understanding Elasticsearch
Elasticsearch is a part of the ELK (Elasticsearch, Logstash, Kibana) Stack, a popular suite of tools used to collect, analyze, and visualize data. Originally designed for log and event data, excels in processing large volumes of text-based information and can handle various types of structured and unstructured data. Its core strengths include:
- Speed: is known for its quick search and retrieval capabilities. It can scale horizontally, making it ideal for processing extensive datasets.
- Full-text Search: With its powerful inverted indexing, Elasticsearch can efficiently perform full-text searches, a vital function for text-heavy applications.
- Analytics: Elasticsearch isn’t just a search engine; it can also be used for complex data aggregation and analytics, allowing users to run sophisticated queries across datasets.

2. Role of Artificial Intelligence
AI technologies, especially machine learning (ML), are redefining how organizations handle data. These systems can process enormous datasets to identify trends, make predictions, or automate decision-making processes. When AI algorithms are applied to search engines like, they can learn from the data’s context, understanding and improving the relevance of results over time.
By using AI, Elasticsearch becomes a smarter tool that can enhance traditional search functions in innovative ways, such as:
- Personalized Search Results: AI can tailor search results to individual users based on their preferences and previous interactions.
- Natural Language Processing (NLP): With NLP can interpret search queries in a way that mirrors human language, making it more intuitive for users.
- Anomaly Detection: ML models integrated into can identify unusual patterns in real-time, which is particularly useful in cybersecurity and fraud prevention.
3. Key Applications of Elasticsearch and AI
E-commerce: Personalized Customer Experiences
In the e-commerce sector, and AI are enhancing product search capabilities. AI algorithms can analyze past customer behavior, predict preferences, and display personalized results. For example:
- Product Recommendations: Based on browsing history, AI-driven recommendations appear alongside regular search results, boosting conversion rates.
- Visual Search: Using AI-powered image recognition, customers can search for products by uploading photos, with Elasticsearch helping to identify and display relevant items.
Healthcare: Real-Time Patient Monitoring and Diagnosis
In healthcare, Elasticsearch and AI can be applied to streamline patient care and operational efficiency. For example:
- Predictive Diagnosis: AI models can analyze patient records and predict potential health risks, which are then quickly accessible through powered search.
- Anomaly Detection in Patient Data: By analyzing real-time data, such as heart rate or oxygen levels, AI models integrated with can detect anomalies, alerting healthcare providers promptly.
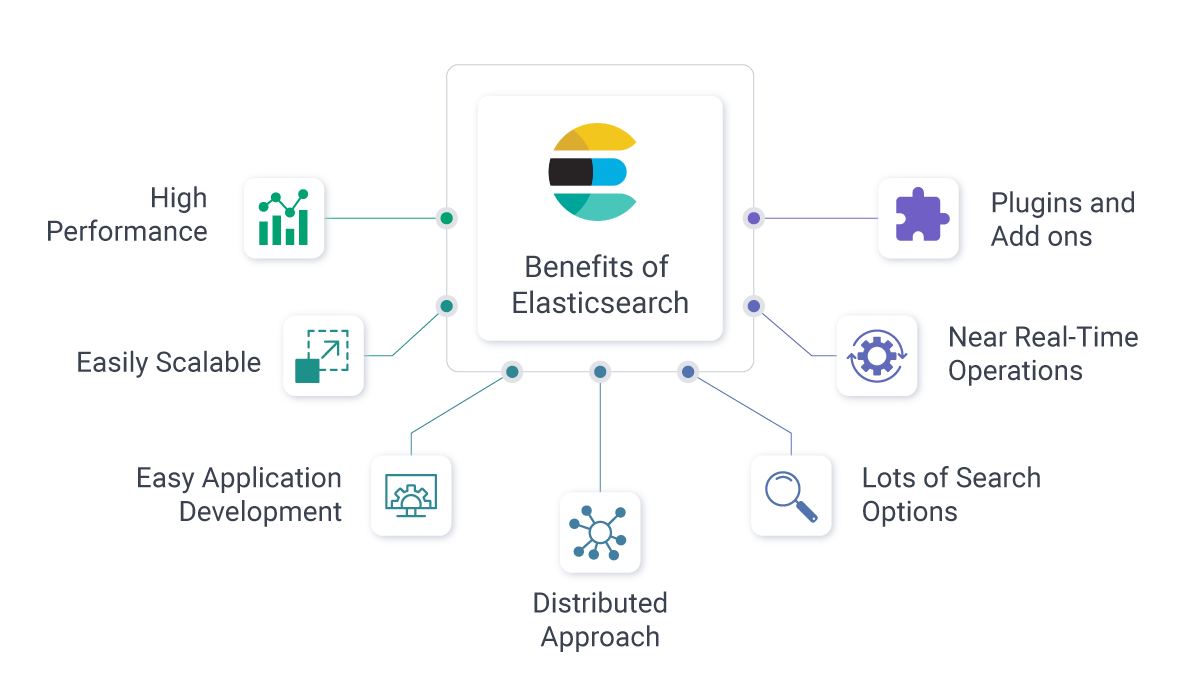
Finance: Fraud Detection and Risk Assessment
Financial institutions are heavily reliant on Elasticsearch and AI for data-driven decisions in areas like fraud detection and risk assessment.
- Risk Prediction Models: AI algorithms analyze transaction histories to assess risk, with Elasticsearch enabling fast, real-time analysis.
- Fraud Detection: Machine learning models can identify abnormal transaction patterns. Elasticsearch facilitates these detections by enabling swift access to past transaction data for comparison.
Cybersecurity: Threat Detection and Response
In cybersecurity, Elasticsearch’s speed and scalability combined with AI’s predictive capabilities make for a formidable tool in threat detection.
- Anomaly Detection in Logs: By analyzing log data in real-time, AI-powered Elasticsearch can identify suspicious patterns, such as repeated login attempts or unusual traffic spikes.
- Automated Responses: Machine learning models can prioritize detected threats, helping security teams focus on the most critical issues.
4. Challenges and Considerations
While Elasticsearch and AI offer significant advantages, there are challenges:
- Data Quality: For AI models to be effective, they need high-quality data. can help filter and organize data, but careful data curation is essential.
- Scalability: As datasets grow, maintaining the efficiency and speed of Elasticsearch becomes more complex. Proper indexing and data structuring are necessary.
- Privacy and Compliance: Particularly in healthcare and finance, organizations need to ensure they’re handling data responsibly and complying with regulations, such as GDPR.
5. Future of Elasticsearch and AI
The integration of Elasticsearch and AI will only deepen as data volumes increase and the demand for intelligent, real-time search intensifies. Innovations such as:
- Semantic Search: AI advancements are making it possible for Elasticsearch to understand the “meaning” behind words, rather than just matching keywords.
- Voice and Visual Search: NLP and computer vision capabilities will enable Elasticsearch to support voice and image-based queries, making search more versatile.
- Real-Time Data Analytics: AI-powered analytics within will allow industries to analyze and act on data instantly, a capability that’s increasingly essential in sectors like IoT and autonomous vehicles.
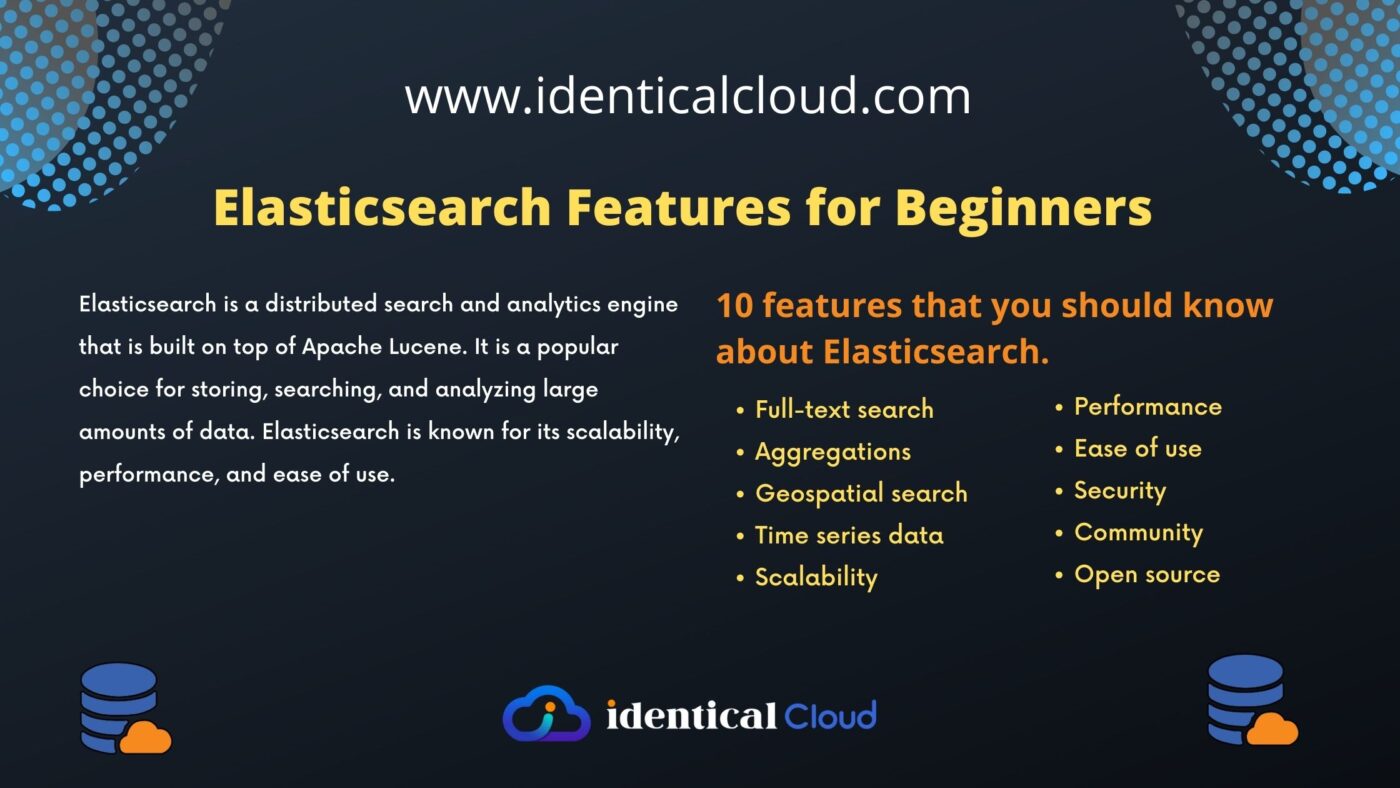
Conclusion
Elasticsearch and artificial intelligence together create powerful, scalable solutions across sectors. By incorporating AI’s learning abilities with fast retrieval and analytics, organizations can make smarter decisions, detect risks proactively, and enhance user experiences. As the two fields evolve, their combined potential will continue to unlock transformative capabilities for data-driven industries.
Q1: What is Elasticsearch, and why is it popular?
A: Elasticsearch is a distributed search and analytics engine designed to quickly process large amounts of data. It’s particularly popular because of its ability to perform fast, full-text searches and handle a variety of structured and unstructured data. is part of the ELK Stack (Elasticsearch, Logstash, Kibana), which many organizations use for log analysis, monitoring, and other data-heavy applications.
Q2: How does artificial intelligence enhance Elasticsearch?
A: AI enhances by making it “smarter” and more responsive to complex queries. For example, AI algorithms can be applied to personalize search results, recognize patterns in data, and perform natural language processing (NLP), which helps the search engine interpret and understand human language. AI-powered Elasticsearch can also detect anomalies in data, improving capabilities in areas like cybersecurity and fraud detection.
Q3: What is the role of Natural Language Processing (NLP) in Elasticsearch?
A: NLP allows Elasticsearch to better understand and interpret the context of search queries. Instead of matching only specific keywords, NLP enables Elasticsearch to process queries in a way that’s closer to human language, which can yield more accurate results. This is particularly useful in applications like customer support and search engines, where users may input questions in a conversational style.
Q4: Can Elasticsearch and AI be used for real-time data analysis?
A: Yes, Elasticsearch combined with AI is highly effective for real-time data analysis. AI models can process incoming data to identify trends, detect anomalies, and make predictions, all in real time. For example, in the healthcare sector, patient monitoring systems can analyze real-time data such as heart rate and alert medical staff if there’s an anomaly. speed and scalability make it an ideal engine for such real-time applications.
Q5: What are some common applications of Elasticsearch and AI in e-commerce?
A: In e-commerce, Elasticsearch and AI are used to personalize shopping experiences and improve product search accuracy. Common applications include:
- Personalized Recommendations: AI algorithms analyze past user behavior to suggest products that users are likely to be interested in.
- Visual Search: Using AI-powered image recognition, customers can search for products by uploading images, and helps to identify similar items.
- Customer Sentiment Analysis: AI can analyze customer reviews to gauge sentiment, and Elasticsearch makes this data quickly accessible to e-commerce platforms.

Q6: How are Elasticsearch and AI used in cybersecurity?
A: In cybersecurity, Elasticsearch and AI work together to enhance threat detection and response. AI algorithms can monitor network logs to detect unusual patterns or activities, like frequent login attempts or unexpected traffic. enables rapid searching and analysis of these logs, helping security teams to spot potential threats in real time and prioritize responses accordingly.
Q7: What challenges can arise when integrating Elasticsearch and AI?
A: Some common challenges include:
- Data Quality: AI models need high-quality data to make accurate predictions, so the data used with Elasticsearch must be well-organized and relevant.
- Scalability: Large datasets can slow down Elasticsearch’s performance. To address this, organizations need to properly structure and index data.
- Privacy and Compliance: Handling sensitive data, especially in fields like healthcare and finance, requires strict adherence to data privacy laws, such as GDPR. Organizations must ensure they’re using data responsibly and securely.
Q8: What are the benefits of using AI for anomaly detection in Elasticsearch?
A: AI-based anomaly detection in Elasticsearch helps identify unusual patterns or events within datasets, often in real time. This is crucial in fields like finance (for fraud detection), cybersecurity (for threat detection), and healthcare (for monitoring patient vitals). AI models can learn normal patterns over time, allowing Elasticsearch to quickly detect deviations and alert relevant teams, which can improve response times and prevent potential issues.
Q9: What industries benefit the most from combining Elasticsearch with AI?
A: While many industries benefit, the ones that see the greatest impact include:
- E-commerce: For personalized search and recommendation systems.
- Healthcare: For predictive diagnostics and real-time patient monitoring.
- Finance: For fraud detection, risk assessment, and compliance.
- Cybersecurity: For anomaly detection and threat response.
profesyonel su kaçak tespiti Şişli’deki evimizde su kaçağını Testo cihazlarıyla hemen buldular, çok hızlı ve profesyonellerdi. https://leenkup.com/ustaelektrikci
Pingback: The Role of Probabilistic Winter Storm Severity Index in 2024 Snow Forecasting". - Deep Learn Daily
Hi there! This post couldn’t be written any better! Reading through this post reminds me of my previous room mate! He always kept talking about this. I will forward this article to him. Pretty sure he will have a good read. Thank you for sharing!
Can you be more specific about the content of your article? After reading it, I still have some doubts. Hope you can help me.
I don’t think the title of your article matches the content lol. Just kidding, mainly because I had some doubts after reading the article.
Thanks for sharing. I read many of your blog posts, cool, your blog is very good.
Your point of view caught my eye and was very interesting. Thanks. I have a question for you.
Thanks for sharing. I read many of your blog posts, cool, your blog is very good.
Can you be more specific about the content of your article? After reading it, I still have some doubts. Hope you can help me.